New thresholding methodology published
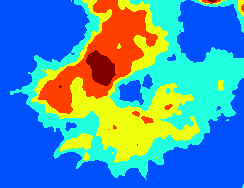
A new methodology for image thresholding has been recently proposed and published in Knowledge Based Systems: "A local fuzzy thresholding methodology for multiregion image segmentation". The methodology overcomes the common drawbacks of thresholding methods when images are corrupted with artifacts and noise. It is based on relating each pixel in the image to different output centroids via a fuzzy membership function, avoiding any initial hard decision.
Abstract: Thresholding is a direct and simple approach to extract different regions from an image. In its basic formulation, thresholding searches for a global value that maximizes the separation between output classes. The use of a single hard threshold value is precisely the source of important segmentation errors in many scenarios like noisy images or uneven illumination. If no connectivity or closed objects are considered, the method is prone to produce isolated pixels. In this paper a new multiregion thresholding methodology is presented to overcome the common drawbacks of thresholding methods when images are corrupted with artifacts and noise. It is based on relating each pixel in the image to different output centroids via a fuzzy membership function, avoiding any initial hard decision. The starting point of the technique is the definition of the output centroids using a clustering method compatible with most thresholding techniques in the literature. The method makes use of the spatial information through a local aggregation step where the membership degree of each pixel is modified by local information that takes into account the memberships of the surrounding pixels. This makes the method robust to noise and artifacts. The general formulation of the proposed methodology allows the design of spatial aggregations for multiple applications, including the possibility of including heuristic information via a fuzzy inference rule base.
Acknowledgements: This work has been partly funded by Ministerio de Ciencia e Innovación under research grant TEC2013-44194 and by the Instituto de Salud Carlos III under Research Grant PI11-01492. A.H. Curiale is funded by the MECyT (Argentina), Fundación Carolina (Spain) and Universidad de Valladolid FPI-Uva. The MATLAB files of the proposed methods can be found at [Mathworks].